See our in-depth guide on AI in drug discovery
Harnessing Genomic and Proteomic Data in Drug Discovery using AI
In the ever-evolving landscape of drug discovery, leveraging cutting-edge AI technologies for drug discovery is of paramount importance. Central to this effort is the utilization of genomic and proteomic data, which provides a wealth of insights into the genetic and protein-level alterations that underpin these diseases. Data collection and preprocessing is the first step in harnessing AI for drug discover. The utilization of genomic and proteomic data is part of the data collection process. This article explores the significance of these data types, the technological advancements enabling their generation, and the integration of this information to drive drug discovery forward.
5/31/20248 min read
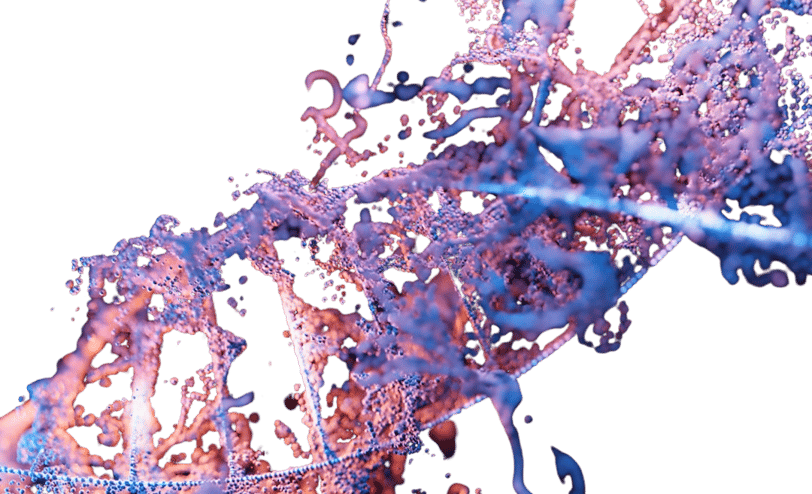
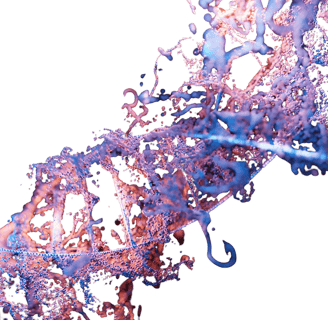
In the ever-evolving landscape of drug discovery, leveraging cutting-edge AI technologies for drug discovery is of paramount importance. Central to this effort is the utilization of genomic and proteomic data, which provides a wealth of insights into the genetic and protein-level alterations that underpin these diseases. Data collection and preprocessing is the first step in harnessing AI for drug discover, as detailed in BioDawn Innovations article Foundations of AI Models in Drug Discovery Series: Step 1 of 6 - Data Collection and Preprocessing in Drug Discovery. The utilization of genomic and proteomic data is part of the data collection process. This article explores the significance of these data types, the technological advancements enabling their generation, and the integration of this information to drive drug discovery forward.
The Power of Genomic Data
Genomic data, which encompasses the complete DNA sequence of an organism, including all its genes, serves as the foundation for understanding biological functions and disease mechanisms. This comprehensive genetic blueprint offers crucial insights into how organisms grow, develop, and respond to environmental factors. Recent advancements in sequencing technologies have significantly enhanced our capacity to generate extensive genomic datasets, propelling forward the fields of genetics, genomics, and personalized medicine. Key sequencing techniques driving these advancements include Whole-Genome Sequencing (WGS), RNA Sequencing (RNA-Seq), and Single-Cell Sequencing.
Whole-Genome Sequencing (WGS)
Whole-Genome Sequencing provides an exhaustive map of an organism's entire genome. This technique sequences all the DNA, identifying every nucleotide that constitutes the genome. By examining the complete genetic code, WGS allows researchers to:
- Identify Genetic Variations: Detect single nucleotide polymorphisms (SNPs), insertions, deletions, and structural variations that may contribute to disease susceptibility and progression.
- Discover Rare Mutations: Uncover mutations that are rare but have significant implications for disease, which might be missed by more targeted sequencing approaches.
- Understand Evolutionary Relationships: Trace evolutionary patterns and relationships between species, as well as within populations, providing insights into genetic diversity and adaptation.
- Facilitate Precision Medicine: Enable the development of personalized treatment plans based on an individual’s unique genetic profile, optimizing therapeutic efficacy and minimizing adverse effects.
WGS has been pivotal in identifying genetic drivers of diseases such as cancer, where mutations in specific genes can lead to uncontrolled cell growth. It also aids in understanding complex genetic disorders, where multiple genetic factors interact to influence disease outcomes.
RNA Sequencing (RNA-Seq)
RNA Sequencing focuses on the transcriptome, the complete set of RNA transcripts produced by the genome under specific conditions. This technique provides insights into gene expression patterns, allowing researchers to:
- Quantify Gene Expression Levels: Measure the abundance of RNA transcripts, revealing which genes are active and to what extent in different tissues or disease states.
- Identify Differential Expression: Compare gene expression profiles between healthy and diseased states to identify genes that are upregulated or downregulated in response to disease.
- Detect Alternative Splicing Events: Uncover different splicing variants of RNA transcripts, which can lead to the production of diverse protein isoforms with varying functions and implications in disease.
- Characterize Non-Coding RNAs: Explore the roles of non-coding RNAs, such as microRNAs and long non-coding RNAs, which regulate gene expression and contribute to disease mechanisms.
RNA-Seq has revolutionized our understanding of dynamic gene regulation and cellular responses. In cancer research, it helps in identifying oncogenes and tumor suppressor genes whose expression levels are altered, providing potential targets for therapy.
Single-Cell Sequencing enables the analysis of genomic information at the single-cell level, offering a detailed view of cellular heterogeneity. This technique allows researchers to:
- Examine Cellular Diversity: Identify distinct cell types within a tissue, revealing the complexity and diversity of cellular populations that might be masked in bulk sequencing approaches.
- Track Cell Lineages: Trace the developmental lineage of individual cells, understanding how they differentiate and contribute to tissue formation and function.
- Study Microenvironments: Investigate the interactions and signaling between different cell types within their native microenvironments, which is particularly relevant in tumor biology.
- Identify Rare Cell Populations: Detect rare cell populations, such as cancer stem cells or immune cells, which play critical roles in disease progression and response to therapy.
Single-cell sequencing has been transformative in oncology, where it aids in understanding tumor heterogeneity, metastasis, and resistance to treatment. By capturing the unique genetic profiles of individual cells, this approach provides a deeper understanding of the cellular mechanisms driving disease.
Integrating Genomic Data in Drug Discovery
The integration of genomic data from WGS, RNA-Seq, and single-cell sequencing into our AI-powered drug discovery platform is instrumental. By leveraging these comprehensive datasets, researchers can:
- Identify Novel Therapeutic Targets: Pinpoint genetic and transcriptional alterations that serve as potential targets for new drugs.
- Predict Drug Responses and Resistance: Anticipate how genetic variations and gene expression profiles influence patient responses to treatments, allowing for more personalized therapeutic strategies.
- Uncover Mechanistic Insights: Decode the molecular pathways and networks involved in disease, providing a foundation for developing more effective and targeted interventions.
The Impact of Proteomic Data
While genomic data provides the blueprint for cellular functions, proteomic data offers a real-time snapshot of the proteins that execute these functions. Proteins, often referred to as the workhorses of the cell, perform a myriad of critical tasks, from catalyzing biochemical reactions to serving as structural components and signaling molecules. Understanding the expression levels, modifications, and interactions of proteins is crucial for deciphering disease mechanisms, especially in complex conditions such as aging and cancer. Proteomic techniques, particularly mass spectrometry, have become indispensable in this field, enabling researchers to delve deeply into the protein landscape. Here, we explore key aspects of proteomics: protein expression profiling, post-translational modifications (PTMs), and protein-protein interactions.
Protein Expression Profiling
Protein expression profiling involves quantifying the abundance of thousands of proteins in a sample simultaneously. This comprehensive approach allows for the identification of proteins that are dysregulated in disease states. Key benefits include:
- Detection of Disease Biomarkers: By comparing protein expression levels between healthy and diseased tissues, researchers can identify biomarkers that signal the presence or progression of a disease. For example, overexpression of certain proteins can indicate cancer.
- Understanding Disease Pathophysiology: Profiling protein expression helps elucidate the molecular mechanisms underlying diseases. This is particularly valuable in complex diseases like cancer, where numerous proteins may be involved in driving malignancy.
- Guiding Therapeutic Development: Identifying dysregulated proteins can reveal new therapeutic targets. Drugs can be designed to modulate the activity or expression of these proteins, offering potential treatments.
Mass spectrometry is a powerful tool for protein expression profiling, allowing for high-throughput and precise quantification of protein levels in diverse biological samples.
Post-Translational Modifications (PTMs)
Post-translational modifications are chemical modifications that occur on proteins after they are synthesized. These modifications can significantly alter protein function, stability, localization, and interactions. Key types of PTMs include phosphorylation, ubiquitination, glycosylation, and acetylation. Understanding PTMs is critical for several reasons:
- Regulation of Protein Function: PTMs can activate or deactivate proteins, thus regulating various cellular processes. For instance, phosphorylation often plays a pivotal role in signaling pathways that control cell growth and division.
- Disease Mechanisms: Aberrant PTMs are implicated in many diseases. In cancer, abnormal phosphorylation of signaling proteins can lead to uncontrolled cell proliferation. Similarly, defective ubiquitination can result in the accumulation of damaged proteins, contributing to neurodegenerative diseases.
- Therapeutic Targeting: PTMs themselves can be targeted therapeutically. Inhibitors of kinases (enzymes that phosphorylate proteins) are a common class of cancer drugs. By understanding PTMs, researchers can develop targeted therapies that modulate these modifications.
Mass spectrometry excels in identifying and characterizing PTMs, providing detailed information on the modification sites and their impact on protein function.
Protein-Protein Interactions
Proteins rarely act alone; they interact with other proteins to form complexes and signaling networks that drive cellular functions. Mapping protein-protein interactions (PPIs) is crucial for several reasons:
- Understanding Cellular Pathways: PPIs help delineate the pathways and networks that orchestrate cellular processes. This knowledge is vital for understanding how cells respond to various stimuli and how these responses are altered in diseases.
- Identifying Key Regulators: Within protein interaction networks, certain proteins serve as key regulators or hubs. These proteins are often critical for maintaining cellular functions and can be potential drug targets.
- Revealing Disease Mechanisms: Changes in PPIs can disrupt cellular homeostasis and lead to disease. For example, in cancer, mutations may alter the interaction between tumor suppressors and oncogenes, promoting tumorigenesis.
Techniques like co-immunoprecipitation, yeast two-hybrid screening, and mass spectrometry are used to identify and characterize PPIs. These interactions provide insights into the functional relationships and pathways involved in aging and cancer.
Integrating Proteomic Data in Drug Discovery
The integration of proteomic data with genomic information creates a holistic view of cellular biology, illuminating the molecular underpinnings of diseases and identifying potential therapeutic targets. At BioDawn Innovations, we leverage this integrated approach to enhance our AI-powered drug discovery platform. By combining proteomic and genomic data, we can:
- Identify Novel Therapeutic Targets: Discover new targets based on both genetic mutations and protein dysregulation.
- Predict Drug Responses: Use proteomic data to understand how protein modifications and interactions affect drug efficacy and resistance.
- Uncover Mechanistic Insights: Decode complex molecular pathways and networks to identify critical nodes that can be targeted for therapeutic intervention.
Meticulous data collection and preprocessing ensures the robustness of the proteomic data used in the drug discovery process. For more information on our foundational data collection and preprocessing, visit our article Foundations of AI Models in Drug Discovery Series: Step 1 of 6 - Data Collection and Preprocessing in Drug Discovery
By harnessing the power of proteomic data, we can advance frontiers of aging and cancer research, paving the way for the development of innovative therapies that improve patient outcomes and quality of life.
Conclusion
The integration of genomic and proteomic data is transforming our understanding of aging and cancer. At BioDawn Innovations, we are committed to leveraging these powerful datasets through advanced AI technologies to accelerate the discovery of novel therapeutics. By unraveling the genetic and protein-level intricacies of these diseases, we aim to pioneer new treatments that improve longevity and quality of life for patients worldwide.
Stay tuned for more insights from our ongoing series as we continue to explore the intersections of AI, genomics, and proteomics in the quest for groundbreaking medical breakthroughs.
References:
1. National Human Genome Research Institute. Whole Genome Sequencing. Available at: https://www.genome.gov/about-genomics/fact-sheets/Whole-Genome-Sequencing-Fact-Sheet.
2. Goodwin, S., McPherson, J. D., & McCombie, W. R. (2016). Coming of age: ten years of next-generation sequencing technologies. Nature Reviews Genetics, 17(6), 333-351.
3. Mortazavi, A., Williams, B. A., McCue, K., Schaeffer, L., & Wold, B. (2008). Mapping and quantifying mammalian transcriptomes by RNA-Seq. Nature Methods, 5(7), 621-628.
4. Stark, R., Grzelak, M., & Hadfield, J. (2019). RNA sequencing: the teenage years. Nature Reviews Genetics, 20(11), 631-656.
5. Tang, F., Barbacioru, C., Wang, Y., Nordman, E., Lee, C., Xu, N., ... & Surani, M. A. (2009). mRNA-Seq whole-transcriptome analysis of a single cell. Nature Methods, 6(5), 377-382.
6. Kolodziejczyk, A. A., Kim, J. K., Svensson, V., Marioni, J. C., & Teichmann, S. A. (2015). The technology and biology of single-cell RNA sequencing. Molecular Cell, 58(4), 610-620.
7. Aebersold, R., & Mann, M. (2003). Mass spectrometry-based proteomics. Nature, 422(6928), 198-207.
8. Zhang, Y., Fonslow, B. R., Shan, B., Baek, M. C., & Yates, J. R. (2013). Protein analysis by shotgun/bottom-up proteomics. Chemical Reviews, 113(4), 2343-2394.
9. Walsh, C. T., Garneau-Tsodikova, S., & Gatto, G. J. (2005). Protein posttranslational modifications: the chemistry of proteome diversifications. Angewandte Chemie International Edition, 44(45), 7342-7372.
10. Jensen, O. N. (2006). Interpreting the protein language using proteomics. Nature Reviews Molecular Cell Biology, 7(6), 391-403.
11. Krogan, N. J., & Cagney, G. (2012). Mapping protein complex interactions using mass spectrometry. Current Opinion in Biotechnology, 23(4), 564-571.
12. Petschnigg, J., Groisman, B., Kotlyar, M., Taipale, M., Zheng, Y., Kurat, C. F., ... & Tyers, M. (2014). The mammalian-membrane two-hybrid assay (MaMTH) for probing membrane-protein interactions in human cells. Nature Methods, 11(5), 585-592.
13. Vamathevan, J., Clark, D., Czodrowski, P., Dunham, I., Ferran, E., Lee, G., ... & Zhao, S. (2019). Applications of machine learning in drug discovery and development. Nature Reviews Drug Discovery, 18(6), 463-477.
14. Mamoshina, P., Vieira, A., Putin, E., & Zhavoronkov, A. (2016). Applications of deep learning in biomedicine. Molecular Pharmaceutics, 13(5), 1445-1454.
15. BioDawn Innovations. (2024). Foundations of AI Models in Drug Discovery Series: Step 1 of 6 - Data Collection and Preprocessing in Drug Discovery. Available at: https://www.biodawninnovations.com/data-collection-and-preprocessing-in-drug-discovery.
BioDawn Innovations' Foundations of AI Models in Drug Discovery Series:
Part 1 of 6 - Data Collection and Preprocessing in Drug Discovery
Part 2 of 6 - Feature Engineering and Selection in Drug Discovery
Part 3 of 6 - Model Selection and Training in Drug Discovery
Part 4 of 6 - Model Evaluation and Validation in Drug Discovery
Part 5 of 6 - Model Interpretation and Deployment in Drug Discovery
Part 6 of 6 - Continuous Improvement and Optimization in Drug Discovery