See our in-depth guide on AI in drug discovery
Semantic Annotation and Metadata Enrichment: Biomedical Data Analysis with AI in Drug Discovery
In the field of data-driven drug discovery and biomedical research, the ability to extract meaningful insights from vast datasets has become a cornerstone of scientific advancement. As the volume of data continues to grow exponentially, so does the challenge of ensuring that this data is accessible, interpretable, and actionable for researchers. One of the most powerful tools in addressing this challenge is the process of semantic annotation and metadata enrichment, which helps contextualize data for enhanced querying, filtering, and semantic inference.
8/16/20245 min read
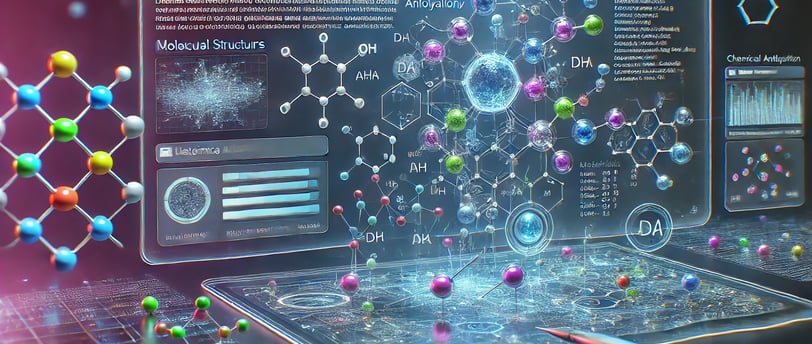
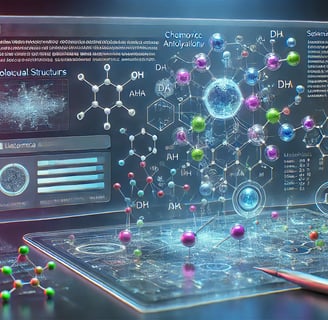
Understanding Semantic Annotation
The Role of Ontologies in Semantic Annotation
Metadata Enrichment: Contextualizing Data for Deeper Insights
Enabling Semantic Inference with Enriched Metadata
Challenges and Considerations
Conclusion
References
1. National Center for Biomedical Ontology. (n.d.). Gene Ontology (GO).
2. Human Aging Genomic Resources. (n.d.). HAGR Database.
3. National Institutes of Health (NIH). (n.d.). Unified Medical Language System (UMLS).
4. BioDawn Innovations. (2024). Foundations of AI Models in Drug Discovery Series: Step 2 of 6 - Feature Engineering and Selection in Drug Discovery.