See our in-depth guide on AI in drug discovery
From Discovery to Market: The Role of AI in the Entire Drug Development Lifecycle
Artificial intelligence (AI) encompasses a diverse set of technologies that enable computers to perform tasks that typically require human intelligence, such as learning from data, recognizing patterns, and making predictions. This article by BioDawn Innovations, presents the transformative impact of artificial intelligence (AI) on the entire drug development lifecycle, from initial discovery to market introduction. It explores how AI accelerates the identification of promising drug candidates, optimizes preclinical testing, enhances the design and execution of clinical trials, streamlines regulatory approval, and supports market access and post-market surveillance. By examining each phase of drug development, the article highlights the ways in which AI technologies are making the process faster, more efficient, and more personalized, ultimately leading to innovative treatments and improved patient outcomes.
5/16/202410 min read
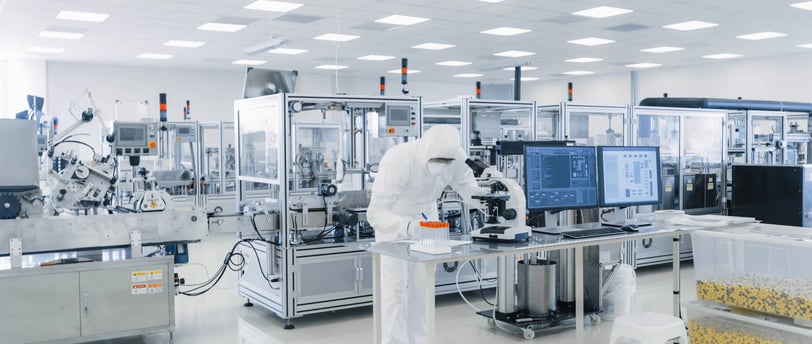
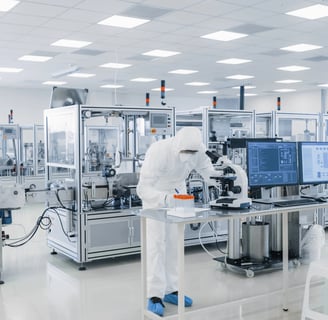
Artificial intelligence (AI) encompasses a diverse set of technologies that enable computers to perform tasks that typically require human intelligence, such as learning from data, recognizing patterns, and making predictions. This article by BioDawn Innovations, presents the transformative impact of artificial intelligence (AI) on the entire drug development lifecycle, from initial discovery to market introduction. It explores how AI accelerates the identification of promising drug candidates, optimizes preclinical testing, enhances the design and execution of clinical trials, streamlines regulatory approval, and supports market access and post-market surveillance. By examining each phase of drug development, the article highlights the ways in which AI technologies are making the process faster, more efficient, and more personalized, ultimately leading to innovative treatments and improved patient outcomes.
1. Drug Discovery and Design
AI accelerates the initial stages of drug discovery by predicting molecular interactions, generating new drug candidates, and screening compounds. Machine learning algorithms can predict how different molecules interact with biological targets by analyzing vast datasets. AI-driven generative models design new drug molecules with desired properties, while high-throughput virtual screening rapidly evaluates large libraries of compounds. Read BioDawn Innovations' Foundations of AI Models in Drug Discovery Series (link)
2. Preclinical Testing
AI aids preclinical testing by significantly enhancing the ability to predict toxicity and side effects, as well as by simulating complex biological processes in a more detailed and accurate manner. During preclinical testing, AI models utilize vast amounts of biological and chemical data to assess the safety profile of drug candidates comprehensively. This involves evaluating the potential interactions between the drug and various biological systems to identify any toxicity issues that could arise. By identifying these potential issues early in the development process, AI helps to mitigate risks, ensuring that only the safest and most promising drug candidates advance to clinical trials, which are both costly and time-consuming.
In addition to predicting toxicity, AI-driven simulations play a crucial role in understanding the pharmacokinetics and pharmacodynamics of a drug. Pharmacokinetics involves predicting how a drug is absorbed, distributed, metabolized, and excreted by the body. AI models can simulate these processes, providing detailed insights into how the drug moves through and affects the body over time. This includes identifying the optimal dosage and delivery method to maximize efficacy while minimizing adverse effects.
Pharmacodynamics, on the other hand, focuses on the biological effects of the drug and its mechanisms of action. AI simulations can predict how the drug interacts with its target sites, such as receptors or enzymes, and how these interactions translate into therapeutic effects or potential side effects. By modeling these interactions, AI helps researchers understand the drug's efficacy and potential impact on various biological pathways.
Moreover, AI can integrate data from various sources, including genomic, proteomic, and metabolomic data, to provide a holistic view of the drug's effects on the body. This integrative approach allows for the identification of biomarkers that can predict individual responses to the drug, paving the way for personalized medicine.
The ability of AI to process and analyze large datasets quickly and accurately also enables the identification of previously unnoticed patterns and correlations. This can lead to the discovery of new drug targets and the repurposing of existing drugs for new therapeutic applications.
By leveraging AI in preclinical testing, researchers can conduct more efficient and precise evaluations of drug candidates. This reduces the likelihood of late-stage failures, accelerates the overall drug development timeline, and ultimately leads to the development of safer and more effective drugs. AI's role in preclinical testing is thus a critical component of modern drug development, driving innovation and improving patient outcomes.
3. Clinical Trials Design and Execution
AI enhances clinical trials by revolutionizing various aspects of trial design, patient recruitment and stratification, and monitoring and data analysis. This integration of AI technologies ensures that clinical trials are more efficient, cost-effective, and capable of delivering reliable results in a shorter timeframe.
Firstly, AI optimizes trial design by analyzing vast amounts of historical trial data and existing medical literature to identify the most effective and efficient trial protocols. This includes determining optimal dosage levels, treatment durations, and patient monitoring schedules. By simulating different trial scenarios, AI can predict potential outcomes and pitfalls, allowing researchers to refine their trial designs before actual implementation. This reduces the likelihood of trial amendments, which can be costly and time-consuming.
In the realm of patient recruitment and stratification, AI plays a pivotal role by leveraging advanced algorithms to sift through electronic health records (EHRs), genetic data, and other patient information to identify individuals who meet the specific inclusion and exclusion criteria for a trial. This targeted approach not only accelerates the recruitment process but also ensures a more homogeneous and relevant participant pool, which is crucial for obtaining statistically significant results. AI can also stratify patients into subgroups based on genetic markers, disease subtypes, and other relevant factors, enabling personalized treatment approaches and more precise evaluations of the drug's efficacy and safety.
Once the trial is underway, AI enhances monitoring and data analysis by providing real-time insights into patient health and trial progress. Continuous monitoring systems powered by AI can detect adverse effects and deviations from expected outcomes more quickly than traditional methods. This allows for prompt intervention, ensuring patient safety and maintaining the integrity of the trial. AI-driven analytics can process vast amounts of trial data in real-time, identifying trends and correlations that might not be immediately apparent to human researchers. This real-time analysis enables adaptive trial designs, where protocols can be modified on-the-fly based on interim results, further improving trial efficiency and effectiveness.
Additionally, AI facilitates the integration and analysis of diverse data sources, including clinical, genomic, imaging, and wearable device data. This holistic approach provides a more comprehensive understanding of the drug's effects and patient responses. For example, AI can analyze data from wearable devices to monitor patient activity levels, heart rate, and other vital signs, providing continuous health insights without the need for frequent clinic visits.
AI also aids in ensuring compliance with regulatory requirements by automating the documentation and reporting processes. It can generate detailed reports and ensure that all necessary data is accurately recorded and submitted to regulatory bodies, reducing the administrative burden on researchers and minimizing the risk of human error.
In summary, AI significantly enhances the efficiency and effectiveness of clinical trials by optimizing trial design, improving patient recruitment and stratification, and providing advanced monitoring and real-time data analysis. These advancements not only accelerate the drug development process but also improve the likelihood of successful outcomes, ultimately leading to the faster introduction of new and effective treatments to the market.
4. Regulatory Approval
AI streamlines the regulatory process by making it more efficient and reliable through advanced data compilation, organization, and predictive analytics. This technology assists pharmaceutical companies in navigating the complex and stringent requirements set forth by regulatory agencies, such as the FDA in the United States and the EMA in Europe.
Firstly, AI helps compile the vast amounts of data required for regulatory submissions. The drug development process generates extensive datasets, including preclinical study results, clinical trial data, pharmacokinetic and pharmacodynamic profiles, manufacturing details, and safety information. AI systems can automatically organize and integrate this diverse data, ensuring that it is comprehensive and well-structured. By utilizing natural language processing (NLP) and machine learning algorithms, AI can extract relevant information from various documents, databases, and reports, compiling them into a coherent submission package. This reduces the time and effort required for manual data handling and minimizes the risk of errors or omissions.
In addition to data compilation, AI plays a crucial role in analyzing the data to ensure it meets regulatory standards. Regulatory bodies have specific guidelines and criteria that must be adhered to, and any deviations can result in delays or rejections. AI tools can cross-reference the compiled data with these guidelines, identifying any discrepancies or areas that need further attention. This proactive approach ensures that the submission is not only complete but also compliant with regulatory requirements, thereby increasing the chances of approval.
Furthermore, AI can predict the likelihood of regulatory approval by analyzing data from past submissions. By examining the outcomes of previous applications, AI models can identify patterns and factors that contribute to successful approvals or rejections. These models can assess various aspects, such as the robustness of clinical trial data, the safety and efficacy profiles of the drug, and the quality of the manufacturing process. By comparing the current submission with historical data, AI can provide insights into potential strengths and weaknesses, allowing companies to address any issues before submitting their application. This predictive capability helps companies make informed decisions and refine their strategies, ultimately improving their chances of regulatory success.
AI's ability to automate and optimize these processes results in significant time and cost savings. The traditional regulatory submission process is labor-intensive and can take months to years to complete. AI accelerates this process by handling repetitive and time-consuming tasks, freeing up human resources to focus on more strategic and complex aspects of the submission. This efficiency is particularly crucial for companies aiming to bring innovative therapies to market quickly, as it reduces the overall time-to-market and allows patients to access new treatments sooner.
Moreover, AI can enhance communication with regulatory bodies throughout the submission process. AI-driven platforms can facilitate real-time updates and interactions, ensuring that any queries or requests for additional information from regulatory agencies are promptly addressed. This continuous engagement helps maintain a transparent and collaborative relationship with regulators, further smoothing the approval process.
In conclusion, AI significantly streamlines the regulatory process by efficiently compiling and organizing data for submissions, ensuring compliance with regulatory standards, and predicting approval outcomes based on historical data. These capabilities not only expedite the regulatory review process but also increase the likelihood of successful approvals, ultimately enabling faster access to new and effective treatments for patients.
5. Market Access and Post-Market Surveillance
AI supports the market introduction and monitoring of new drugs by leveraging advanced analytics and data-driven insights to optimize various aspects of the process. This includes analyzing market trends, developing market entry strategies, personalizing treatments, and conducting post-market surveillance. By harnessing the power of AI, pharmaceutical companies can make informed decisions, enhance patient outcomes, and ensure the long-term success of their products.
Firstly, AI plays a crucial role in analyzing market trends and patient demographics. By processing large datasets from various sources, including healthcare databases, social media, and market research reports, AI can identify emerging trends, unmet medical needs, and potential opportunities for new drug introductions. This analysis helps companies understand the competitive landscape, forecast demand, and tailor their marketing strategies to target specific patient populations. AI can also segment the market based on demographic factors such as age, gender, geographic location, and socioeconomic status, providing a detailed understanding of different patient groups and their needs.
Developing effective market entry strategies is another key area where AI excels. AI models can simulate different market scenarios, assess potential risks and benefits, and optimize pricing and distribution strategies. For instance, AI can predict how changes in pricing, promotional activities, or distribution channels might impact sales and market share. By integrating real-time data and historical sales information, AI helps companies make data-driven decisions to maximize their market penetration and profitability. Additionally, AI can identify key opinion leaders (KOLs) and influencers within the medical community who can advocate for the new drug, enhancing its acceptance and adoption.
Personalizing treatments is a significant advancement enabled by AI. By analyzing genetic, environmental, and lifestyle factors, AI can identify patient subgroups that are most likely to benefit from a particular drug. This personalized approach, often referred to as precision medicine, ensures that treatments are tailored to individual patients' unique characteristics, improving therapeutic outcomes and reducing adverse effects. For example, AI can analyze genomic data to identify biomarkers that predict a patient’s response to a drug, allowing for the selection of the most effective treatment regimen. This not only enhances patient satisfaction but also increases the likelihood of successful treatment outcomes.
Conducting post-market surveillance is critical for monitoring the safety and efficacy of drugs once they are on the market. AI facilitates continuous monitoring by analyzing real-world data from electronic health records (EHRs), patient registries, adverse event reports, and other sources. This ongoing surveillance helps detect rare or long-term side effects that may not have been evident during clinical trials. AI can quickly identify patterns and correlations in adverse event data, enabling prompt interventions and regulatory actions if needed. Furthermore, AI-powered tools can track patient adherence to treatment regimens, monitor drug performance in diverse populations, and provide insights into the drug’s real-world effectiveness.
Moreover, AI can enhance pharmacovigilance efforts by automating the detection and reporting of adverse events. Natural language processing (NLP) algorithms can scan medical literature, social media, and other unstructured data sources for mentions of adverse reactions, providing a comprehensive view of the drug’s safety profile. This proactive approach to safety monitoring helps maintain public trust and ensures that any potential issues are addressed swiftly.
In summary, AI supports the market introduction and monitoring of new drugs by analyzing market trends and patient demographics, developing data-driven market entry strategies, personalizing treatments based on genetic and lifestyle factors, and conducting robust post-market surveillance. These capabilities not only optimize the launch and uptake of new drugs but also ensure their ongoing safety and efficacy, ultimately improving patient outcomes and fostering innovation in the pharmaceutical industry.
Conclusion
Artificial intelligence (AI) is revolutionizing the drug development lifecycle, enhancing efficiency, accuracy, and effectiveness from discovery to market. AI aids in drug discovery by predicting molecular interactions, generating new drug candidates, and screening compounds. In preclinical testing, AI models predict toxicity and simulate biological processes, providing detailed insights into pharmacokinetics and pharmacodynamics. AI optimizes clinical trials by designing efficient protocols, recruiting suitable participants, and providing real-time monitoring and data analysis. For regulatory approval, AI streamlines data compilation, ensures compliance, and predicts approval outcomes based on historical data. AI also supports market introduction by analyzing market trends, developing entry strategies, personalizing treatments, and conducting post-market surveillance to track drug performance and ensure safety and efficacy.
References:
1. Drug Discovery and Design
"Artificial intelligence for drug discovery, biomarker development, and generation of novel chemistry." (Article by Jensen JH, et al. in Molecular Pharmaceutics, 2020)
"Deep learning in drug discovery and medicine; Scratching the surface." (Article by Schneider P. in Molecular Informatics, 2020)
"Artificial intelligence for drug discovery." (Review by Ghosh S, et al. in Biomolecules, 2021)
2. Preclinical Testing
"Artificial intelligence in preclinical drug development." (Review by Roy P, et al. in Drug Discovery Today, 2020)
"Artificial intelligence in drug discovery: a comprehensive review and multimodal, multi-Omics-based (MMOB) approach for optimizing the success rate of clinical trials in the pharmaceutical industry." (Review by Mamoshina P, et al. in Expert Opinion on Drug Discovery, 2018)
"Artificial intelligence in preclinical drug development: Beyond computational models and automated laboratory technologies." (Article by Pereira JC, et al. in Pharmacological Research, 2021)
3. Clinical Trials Design and Execution
"Artificial intelligence in clinical trial design." (Review by Venkatakrishnan K, et al. in Journal of Personalized Medicine, 2020)
"Artificial intelligence for clinical trial design: a primer." (Article by Johnson KW, et al. in Clinical Pharmacology & Therapeutics, 2021)
"The use of artificial intelligence in the design and conduct of clinical trials." (Review by Debray TPA, et al. in Expert Review of Clinical Pharmacology, 2021)
4. Regulatory Approval
"Artificial intelligence in regulatory decision-making." (Article by Suvarna V, et al. in Bio-IT World, 2020)
"Artificial intelligence in regulatory science: leveraging the full potential of real-world data." (Article by Hirsch G, et al. in Clinical Pharmacology & Therapeutics, 2021)
"Regulatory considerations for artificial intelligence and machine learning technologies in the life sciences." (Article by Woodcock J, et al. in Nature Biotechnology, 2021)
5. Market Access and Post-Market Surveillance
"AI for health: from discovery to adoption." (Article by Topol EJ. in NPJ Digital Medicine, 2019)
"AI in drug development: shifting focus from R&D to market access." (Article by Krishnan A. in Applied Clinical Trials, 2021)
"Real-world data and artificial intelligence for pharmacovigilance: challenges and opportunities." (Article by Härmark L, et al. in Pharmaceutical Medicine, 2021)
BioDawn Innovations' Foundations of AI Models in Drug Discovery Series:
Part 1 of 6 - Data Collection and Preprocessing in Drug Discovery
Part 2 of 6 - Feature Engineering and Selection in Drug Discovery
Part 3 of 6 - Model Selection and Training in Drug Discovery
Part 4 of 6 - Model Evaluation and Validation in Drug Discovery
Part 5 of 6 - Model Interpretation and Deployment in Drug Discovery
Part 6 of 6 - Continuous Improvement and Optimization in Drug Discovery