See our in-depth guide on AI in drug discovery
Feature Discovery in Machine Learning for Drug Discovery
The pharmaceutical industry is at the cusp of a revolution, driven by advancements in artificial intelligence (AI) and machine learning (ML). One of the most promising areas within this revolution is feature discovery in machine learning, which plays a crucial role in accelerating drug discovery. BioDawn Innovations aims to leverage these cutting-edge technologies to unlock new potentials in drug development, enhancing both efficiency and accuracy in identifying promising drug candidates.
6/8/20243 min read
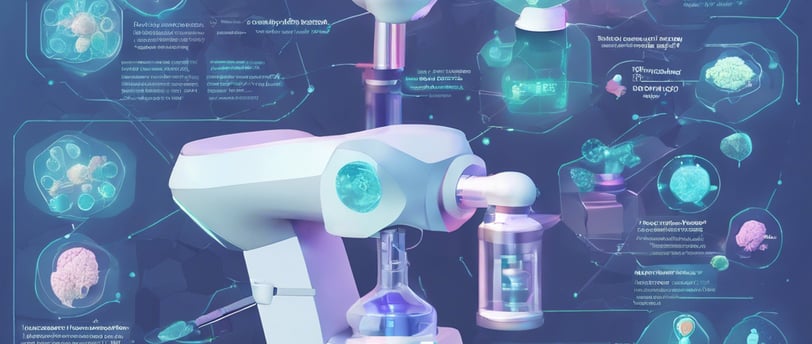
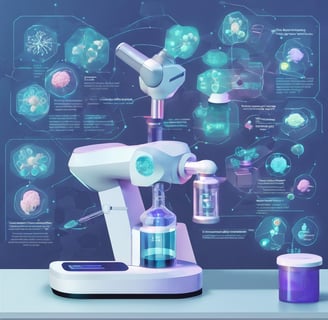
The pharmaceutical industry is at the cusp of a revolution, driven by advancements in artificial intelligence (AI) and machine learning (ML). One of the most promising areas within this revolution is feature discovery in machine learning, which plays a crucial role in accelerating drug discovery. BioDawn Innovations aims to leverage these cutting-edge technologies to unlock new potentials in drug development, enhancing both efficiency and accuracy in identifying promising drug candidates.
What is Feature Discovery?
Feature discovery is a subset of machine learning that involves identifying the most relevant variables (or features) from raw data that are instrumental in predicting outcomes. In the context of drug discovery, these features can be molecular properties, genetic markers, or biochemical interactions that influence the efficacy and safety of potential drugs.
The Importance of Feature Discovery in Drug Discovery
1. Improved Predictive Models:
Accurate feature discovery enables the creation of robust predictive models. By identifying the most significant features, models can more accurately predict how new compounds will behave in biological systems, leading to the identification of promising drug candidates more efficiently.
2. Reduction of Dimensionality:
Biological data is inherently high-dimensional, with numerous variables potentially influencing outcomes. Feature discovery helps reduce this dimensionality, focusing on the most informative variables and thereby simplifying the data without losing critical information. This simplification is crucial for creating more manageable and interpretable models.
3. Enhanced Interpretability:
Understanding which features are most predictive allows scientists to gain insights into the underlying biological mechanisms. This knowledge not only aids in drug discovery but also in understanding disease pathways, which can lead to the identification of new therapeutic targets.
4. Cost and Time Efficiency:
By focusing on the most promising features early in the drug discovery process, researchers can significantly reduce the time and cost associated with experimental validation. This efficiency is particularly vital given the high costs and lengthy timelines traditionally associated with bringing new drugs to market.
Techniques for Feature Discovery
Several advanced techniques are employed in feature discovery for drug discovery:
1. Deep Learning:
Deep learning models, particularly convolutional neural networks (CNNs) and recurrent neural networks (RNNs), have shown great promise in feature discovery. These models automatically learn hierarchical representations of data, capturing complex patterns and interactions that might be missed by traditional methods.
2. Random Forests and Ensemble Methods:
These methods are useful for identifying important features by constructing multiple decision trees and averaging their predictions. The importance of each feature is assessed based on how much it improves the model's predictive accuracy.
3. Principal Component Analysis (PCA):
PCA is a dimensionality reduction technique that transforms high-dimensional data into a lower-dimensional form while preserving as much variance as possible. This technique helps in identifying the most critical features contributing to the variability in the data.
4. Genetic Algorithms:
Inspired by the process of natural selection, genetic algorithms iteratively evolve a population of solutions to optimize feature sets. This approach is particularly useful for exploring large and complex feature spaces.
Innovative Approach
Researchers can integrate these advanced feature discovery techniques into there workflows and this approach involves:
1. Data Integration:
Combining diverse datasets, including genomic, proteomic, and chemical data, to create a comprehensive view of potential drug candidates.
2. Automated Feature Engineering:
Using AI algorithms to automatically generate and select the most relevant features from complex biological data.
3. Continuous Learning:
Implementing continuous learning systems that improve feature discovery models over time as new data becomes available.
4. Collaborative Insights:
Working closely with biologists and chemists to ensure that the features identified are not only statistically significant but also biologically meaningful.
Conclusion
Feature discovery in machine learning is revolutionizing drug discovery by enabling more accurate predictions, reducing costs, and uncovering new biological insights. BioDawn Innovations is at the forefront of this transformation, leveraging state-of-the-art AI and ML techniques to accelerate the development of life-saving drugs. As we continue to refine our methodologies and integrate new data, the potential for breakthroughs in medicine grows exponentially.
References:
- Chen, H., Engkvist, O., Wang, Y., Olivecrona, M., & Blaschke, T. (2018). The rise of deep learning in drug discovery. Drug Discovery Today, 23(6), 1241-1250.
- Brown, N., & Fiscella, M. (2016). The use of machine learning for the prediction of molecular properties and bioactivity. Current Opinion in Chemical Biology, 34, 24-31.
- LeCun, Y., Bengio, Y., & Hinton, G. (2015). Deep learning. Nature, 521(7553), 436-444.
- Breiman, L. (2001). Random forests. Machine Learning, 45(1), 5-32.
- Jolliffe, I.T., & Cadima, J. (2016). Principal component analysis: a review and recent developments. Philosophical Transactions of the Royal Society A: Mathematical, Physical and Engineering Sciences, 374(2065), 20150202.
BioDawn Innovations' Foundations of AI Models in Drug Discovery Series:
Part 1 of 6 - Data Collection and Preprocessing in Drug Discovery
Part 2 of 6 - Feature Engineering and Selection in Drug Discovery
Part 3 of 6 - Model Selection and Training in Drug Discovery
Part 4 of 6 - Model Evaluation and Validation in Drug Discovery
Part 5 of 6 - Model Interpretation and Deployment in Drug Discovery
Part 6 of 6 - Continuous Improvement and Optimization in Drug Discovery