See our in-depth guide on AI in drug discovery
Ensuring Data Integrity and Validity in AI-Driven Drug Discovery
The advent of artificial intelligence (AI) in drug discovery has heralded a new era of biomedical innovation, characterized by the ability to sift through vast datasets to uncover novel therapeutic candidates. Central to this transformative process is the imperative of ensuring data integrity and validity. High-quality data serves as the bedrock upon which AI models build their predictions and insights, making robust data management practices indispensable.
6/3/202410 min read
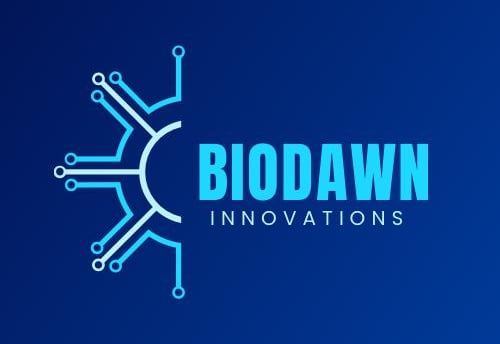
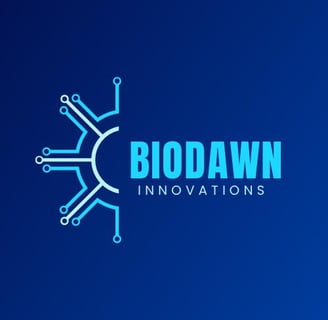
The advent of artificial intelligence (AI) in drug discovery has heralded a new era of biomedical innovation, characterized by the ability to sift through vast datasets to uncover novel therapeutic candidates with unprecedented speed and accuracy. This transformative capability is revolutionizing the pharmaceutical industry, enabling researchers to identify potential drugs more efficiently and at a lower cost than traditional methods. Central to this groundbreaking process is the imperative of ensuring data integrity and validity. High-quality data serves as the bedrock upon which AI models build their predictions and insights, making robust data management practices indispensable. Ensuring the accuracy, consistency, and reliability of data is crucial, as even minor errors or inconsistencies can significantly impact the outcomes of AI-driven research. Therefore, stringent protocols for data collection, validation, and maintenance are essential to harness the full potential of AI in drug discovery, driving the development of new therapies and advancing personalized medicine.
The Importance of Data Integrity in AI-Driven Drug Discovery
Data integrity refers to the accuracy, consistency, and reliability of data throughout its lifecycle. In the context of AI-driven drug discovery, data integrity is paramount for several reasons:
1. Accurate Predictions: AI algorithms rely on historical data to predict outcomes. Any compromise in data quality can lead to erroneous predictions, potentially derailing entire research projects.
2. Regulatory Compliance: The pharmaceutical industry is heavily regulated. Maintaining data integrity ensures compliance with regulations such as Good Laboratory Practices (GLP) and Good Clinical Practices (GCP).
3. Reproducibility: Scientific research mandates that results be reproducible. High data integrity guarantees that other researchers can replicate findings, thereby validating the results.
Selecting Reliable Data Sources
Researchers must exercise judicious discernment in selecting data sources. The selection process should prioritize reliability, relevance, and quality, ensuring the data is fit for purpose. Here are key considerations:
Source Reliability
1. Established Databases:
- Biomedical Databases: Trusted biomedical databases such as PubMed, GenBank, and the European Bioinformatics Institute (EBI) provide peer-reviewed and curated data. These databases are maintained by reputable institutions and are regularly updated, ensuring data reliability.
- Clinical Trial Repositories: ClinicalTrials.gov and the World Health Organization’s International Clinical Trials Registry Platform (ICTRP) offer comprehensive data on clinical trials worldwide. These sources are essential for accessing trial results and protocols, ensuring the data's credibility.
2. Peer-Reviewed Journals:
- High-Impact Journals: Publications in high-impact, peer-reviewed journals are typically subjected to rigorous review processes. Journals such as Nature, Science, and The New England Journal of Medicine provide high-quality research data that can be trusted.
- Reproducibility: Preference should be given to studies that have demonstrated reproducibility. Reliable sources often include supplementary data and detailed methodologies, enabling other researchers to validate findings.
3. Institutional Data:
- Academic Institutions: Data from leading academic institutions and research centers are often at the forefront of scientific discovery. Collaborations with universities and research hospitals can yield high-quality data from well-conducted studies.
- Government Agencies: Agencies such as the National Institutes of Health (NIH), the Food and Drug Administration (FDA), and the European Medicines Agency (EMA) provide extensive datasets from regulatory submissions, surveillance, and funded research projects.
Relevance to Research
1. Specificity to Research Questions:
- Targeted Data: The data should directly relate to the specific biological targets, disease states, or therapeutic areas being investigated. This ensures that the insights derived are directly applicable to the research objectives.
- Contextual Appropriateness: Consider the biological, clinical, and demographic context of the data. Data from studies involving similar populations, disease stages, and conditions are more likely to be relevant and useful.
2. Temporal Relevance:
- Recent Data: In rapidly evolving fields like drug discovery, recent data is often more relevant. Researchers should prioritize the most current studies and datasets to ensure they are working with the latest information and trends.
- Historical Data for Trend Analysis: While recent data is crucial, historical data can provide valuable context for trend analysis and longitudinal studies. Combining both can offer a comprehensive view of the research landscape.
3. Cross-Disciplinary Relevance:
- Interdisciplinary Data Sources: Incorporating data from various disciplines (e.g., genomics, proteomics, pharmacology) can enrich the research. Cross-disciplinary data integration helps in constructing a holistic understanding of complex biological systems.
Data Quality
1. Completeness:
- Comprehensive Datasets: High-quality data should be complete, with minimal missing values. Datasets should include all necessary variables and metadata to support thorough analysis.
- Detailed Annotations: Well-annotated data provides clear descriptions of variables, methodologies, and any preprocessing steps taken, facilitating better understanding and reproducibility.
2. Accuracy:
- Validation and Verification: Data accuracy can be ensured through validation against known standards or through cross-verification with multiple sources. Methods such as experimental replication and independent review are essential.
- Error Rates: Researchers should be aware of the error rates and uncertainties associated with their data sources. Low error rates are indicative of high-quality data.
3. Timeliness:
- Updated Information: Regularly updated data sources reflect the most current research and clinical findings. For instance, genomic databases that are frequently updated with new sequences and annotations are more valuable.
- Data Currency: In fields like pharmacovigilance, up-to-date adverse event reports are crucial for ongoing drug safety monitoring.
4. Provenance:
- Source Traceability: Knowing the origin of the data, including who collected it, how it was collected, and under what conditions, is vital. Detailed provenance information ensures that the data can be trusted and its context understood.
- Ethical Considerations: Data should be sourced ethically, with proper consent from participants and adherence to privacy regulations. Ethical sourcing reinforces the integrity and societal trust in the research.
Robust Data Extraction Protocols
Once reliable data sources are identified, robust data extraction protocols must be implemented to ensure the fidelity of the data being harvested. Key steps include:
1. Automated Extraction Tools: Utilize advanced tools and algorithms for data extraction to minimize human error. These tools can parse large datasets quickly and accurately.
2. Standardization: Ensure data is standardized, making it easier to compare and combine datasets from different sources. This includes harmonizing units of measurement, terminology, and formats.
3. Metadata Documentation: Maintain comprehensive metadata records to provide context about the data. This includes details on data origin, extraction methods, and any transformations applied.
Stringent Validation Procedures
Validation is critical to ensuring the trustworthiness of collected data. Stringent validation procedures involve multiple layers of checks and balances:
1. Cross-Verification: Cross-verify data with multiple sources when possible. This helps identify and rectify discrepancies early in the process.
2. Statistical Validation: Employ statistical techniques to validate the data. Outlier detection, consistency checks, and trend analysis are common methods to identify anomalies.
3. Quality Audits: Regularly conduct data quality audits to ensure ongoing adherence to data integrity standards. This involves systematic reviews of data collection, storage, and processing practices.
The Role of Data Collection in Drug Discovery
Data collection is the genesis of the data-driven drug discovery journey. It is through meticulous curation and discerning selection that researchers lay the groundwork for transformative advancements. Here’s how effective data collection fosters innovation:
1. Understanding Disease Pathology: Comprehensive data collection illuminates the molecular intricacies of disease pathology. By understanding the underlying mechanisms, researchers can identify potential therapeutic targets.
2. Identifying Therapeutic Candidates: High-quality data enables the identification of promising therapeutic candidates. AI algorithms can analyze vast datasets to pinpoint molecules with the potential to become effective treatments.
3. Precision Medicine: Data collection supports the development of precision medicine. By integrating diverse data types (genomic, proteomic, clinical), researchers can tailor treatments to individual patient profiles, enhancing efficacy and reducing adverse effects.
Practical Considerations for Data Integrity and Validity
To effectively ensure data integrity and validity, researchers and organizations should adopt several practical measures. These measures encompass robust governance frameworks, training programs, advanced technological infrastructure, and collaborative efforts, all aimed at safeguarding the accuracy, consistency, and reliability of data.
Governance Frameworks
1. Establish Data Governance Policies:
- Roles and Responsibilities: Clearly define roles and responsibilities for data management within the organization. Designate data stewards who are responsible for maintaining data quality and integrity.
- Standard Operating Procedures (SOPs): Develop and enforce SOPs for data collection, storage, processing, and sharing. SOPs ensure consistency and adherence to best practices across the organization.
2. Data Quality Standards:
- Quality Metrics: Implement quality metrics and benchmarks for evaluating data integrity. Metrics such as completeness, accuracy, and timeliness should be routinely monitored.
- Audit Trails: Maintain detailed audit trails that document all data-related activities. Audit trails enhance transparency and accountability, allowing for the tracking of data changes and user actions.
3. Regulatory Compliance:
- Adherence to Regulations: Ensure compliance with regulatory standards such as Good Laboratory Practices (GLP), Good Clinical Practices (GCP), and data protection laws like GDPR and HIPAA. Regular compliance audits help mitigate the risk of regulatory breaches.
- Ethical Guidelines: Implement ethical guidelines for data collection and usage. This includes obtaining informed consent from study participants and ensuring data anonymization to protect privacy.
Training and Awareness
1. Continuous Education Programs:
- Training Modules: Develop comprehensive training modules that cover data integrity principles, data management best practices, and the use of relevant tools and technologies.
Training and Awareness
1. Continuous Education Programs:
- Training Modules: Develop comprehensive training modules that cover data integrity principles, data management best practices, and the use of relevant tools and technologies. Training should be mandatory for all personnel involved in data handling.
- Certifications: Encourage staff to obtain certifications in data management and related fields. Certified professionals bring a higher level of expertise and commitment to maintaining data quality.
2. Awareness Campaigns:
- Regular Updates: Conduct regular awareness campaigns to keep staff informed about the latest developments, challenges, and best practices in data integrity. This includes updates on new tools, regulatory changes, and emerging threats.
3.Cultural Emphasis:
Foster a culture of data integrity within the organization. Emphasize the importance of data quality in all communications and reward adherence to data integrity practices.
Technology Infrastructure
1. Secure Data Storage:
- Data Repositories: Utilize secure, centralized data repositories for storing research data. Repositories should support version control and allow for secure access and retrieval.
- Encryption: Implement encryption for data at rest and in transit to protect sensitive information from unauthorized access and breaches.
2. Data Management Tools:
- Data Cleaning Software: Employ advanced data cleaning tools to identify and rectify errors, inconsistencies, and missing values in datasets. Automated cleaning processes reduce the risk of human error.
- Data Integration Platforms: Use data integration platforms to harmonize data from disparate sources. Integration tools ensure that data is consistent and standardized, facilitating seamless analysis.
3. Real-Time Monitoring Systems:
- Automated Monitoring: Deploy automated systems for real-time monitoring of data quality. These systems can flag anomalies, detect data corruption, and alert relevant personnel for immediate action.
- Performance Dashboards: Create performance dashboards that provide visual insights into data quality metrics. Dashboards enable continuous tracking and quick identification of potential issues.
Collaborative Efforts
1. Cross-Disciplinary Collaboration:
- Interdisciplinary Teams: Form interdisciplinary teams that include data scientists, biostatisticians, clinicians, and regulatory experts. Collaborative efforts enhance the robustness of data validation processes.
- Knowledge Sharing: Encourage knowledge sharing through regular meetings, workshops, and conferences. Collaborative environments foster the exchange of best practices and innovative solutions.
2. External Partnerships:
- Industry Consortia: Participate in industry consortia and initiatives such as the Innovative Medicines Initiative (IMI) and the Open Targets consortium. These partnerships provide access to shared data resources and collaborative research opportunities.
- Academic Collaborations: Establish partnerships with academic institutions for joint research projects. Academic collaborations can provide access to high-quality data and cutting-edge methodologies.
3. Standardization Initiatives:
- Data Standards: Advocate for and adopt industry-wide data standards such as the Clinical Data Interchange Standards Consortium (CDISC) and the Health Level Seven International (HL7) standards. Standardization ensures data interoperability and enhances collaborative research efforts.
- Best Practices Guidelines: Contribute to the development of best practices guidelines for data integrity. Sharing insights and experiences helps to establish robust data management practices across the industry.
Implementing these Practical Measures
Implementing these practical measures requires a systematic approach and commitment from all levels of the organization:
1. Policy Development and Enforcement:
- Develop detailed policies that encapsulate all aspects of data integrity and validity. Ensure these policies are well-communicated and enforced through regular audits and reviews.
2. Investment in Technology and Training:
- Allocate resources for the procurement of advanced data management tools and technologies. Similarly, invest in continuous training and development programs to enhance staff capabilities in data handling.
3. Fostering a Culture of Integrity:
- Promote a culture where data integrity is viewed as a core value. Recognize and reward individuals and teams who demonstrate exemplary commitment to maintaining data quality.
4. Continuous Improvement:
- Regularly review and update data management practices to keep pace with technological advancements and regulatory changes. Continuous improvement ensures that the organization remains at the forefront of data integrity.
By adopting these practical measures, researchers and organizations can effectively ensure the integrity and validity of their data. This not only enhances the reliability of AI-driven drug discovery but also fosters innovation and accelerates the development of new therapeutic interventions.
References:
1. National Center for Biotechnology Information (NCBI). PubMed. Available at: https://pubmed.ncbi.nlm.nih.gov/ (Accessed: 3 June 2024).
2. National Center for Biotechnology Information (NCBI). GenBank. Available at: https://www.ncbi.nlm.nih.gov/genbank/ (Accessed: 3 June 2024).
3. European Bioinformatics Institute (EBI). Available at: https://www.ebi.ac.uk/ (Accessed: 3 June 2024).
4. ClinicalTrials.gov. Available at: https://clinicaltrials.gov/ (Accessed: 3 June 2024).
5. World Health Organization (WHO). International Clinical Trials Registry Platform (ICTRP). Available at: https://www.who.int/clinical-trials-registry-platform (Accessed: 3 June 2024).
6. Nature Publishing Group. Nature. Available at: https://www.nature.com/ (Accessed: 3 June 2024).
7. American Association for the Advancement of Science (AAAS). Science. Available at: https://www.sciencemag.org/ (Accessed: 3 June 2024).
8. Massachusetts Medical Society. The New England Journal of Medicine. Available at: https://www.nejm.org/ (Accessed: 3 June 2024).
9. National Institutes of Health (NIH). Available at: https://www.nih.gov/ (Accessed: 3 June 2024).
10. U.S. Food and Drug Administration (FDA). Available at: https://www.fda.gov/ (Accessed: 3 June 2024).
11. European Medicines Agency (EMA). Available at: https://www.ema.europa.eu/ (Accessed: 3 June 2024).
12. U.S. Food and Drug Administration. Good Laboratory Practice (GLP) Regulations. Available at: https://www.fda.gov/science-research/field-science/good-laboratory-practice-glp-regulations (Accessed: 3 June 2024).
13. International Council for Harmonisation of Technical Requirements for Pharmaceuticals for Human Use (ICH). Good Clinical Practice (GCP). Available at: https://ichgcp.net/ (Accessed: 3 June 2024).
14. European Commission. General Data Protection Regulation (GDPR). Available at: https://ec.europa.eu/info/law/law-topic/data-protection_en (Accessed: 3 June 2024).
15. U.S. Department of Health and Human Services. Health Insurance Portability and Accountability Act (HIPAA). Available at: https://www.hhs.gov/hipaa/index.html (Accessed: 3 June 2024).
16. Clinical Data Interchange Standards Consortium (CDISC). Available at: https://www.cdisc.org/ (Accessed: 3 June 2024).
17. Health Level Seven International (HL7). Available at: https://www.hl7.org/ (Accessed: 3 June 2024).
18. Innovative Medicines Initiative (IMI). Available at: https://www.imi.europa.eu/ (Accessed: 3 June 2024).
19. Open Targets. Available at: https://www.opentargets.org/ (Accessed: 3 June 2024).
BioDawn Innovations' Foundations of AI Models in Drug Discovery Series:
Part 1 of 6 - Data Collection and Preprocessing in Drug Discovery
Part 2 of 6 - Feature Engineering and Selection in Drug Discovery
Part 3 of 6 - Model Selection and Training in Drug Discovery
Part 4 of 6 - Model Evaluation and Validation in Drug Discovery
Part 5 of 6 - Model Interpretation and Deployment in Drug Discovery
Part 6 of 6 - Continuous Improvement and Optimization in Drug Discovery